Getting started
Last updated: 2020-08-25

I’ll be using this blog to share some general ideas, how-tos and projects. To stay tuned, feel free to subscribe to the RSS feed.
My first blog post will be on ideation of a data science project.
Introduction
Let’s assume you are a business owner. You have heard good things about utilizing data for automation and decision making, but you’re not sure how this could apply to your business. What you need are project ideas. Ideally, you’ll assemble a group of people representing business, IT/ data engineering and data science and set up a workshop to brainstorm.
- business: identifies business problems and defines metrics for success
- data scientists: propose solutions
- IT/ data engineer: makes sure the solutions are feasible in production
To give you a leg up before you actually get to the workshop, check out this excellent checklist and then let’s review some types of data that many businesses have at their disposal [1] and some generic use cases.
Data sources
When you want to start a data driven project, it makes sense to first look at what data you have. Probably you have accumulated several legacy systems over the years - e.g. at some point you started using Customer Relationship Software. The design decisions taken back then still are relevant. They determine what customer data you gather and what structure that data has. The same goes for the other legacy systems - they were built for a specific business purpose, not for data management. This makes sense and it’s fine, just something to keep in mind going forward.
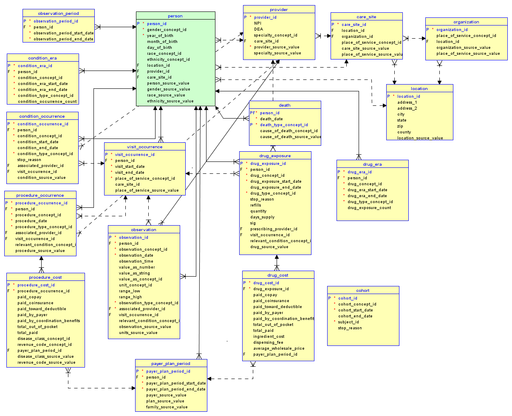
In the following, you can find an overview of data sources and for every source a more detailed explanation.
Examples are fabricated and for illustrative purposes only.
Use cases
Recommendation
Recommendation can: increase revenue by suggesting products to customers they wouldn’t have thought to look for. Or, decrease loss of revenue due to customers who are looking for something you actually offer but can’t find it. Or even be a search engine that increases employee productivity by recommending relevant company wiki articles.
Recommendation is more than an opportunity for a business to increase sales - it can be a real convenience for customers (or employees), even if it’s as simple as “you’re buying a screw, maybe you need a wall plug”.
The key is other peoples’ transactions, be it customer purchases or employee’s access log to wiki articles. You’re leveraging the effort people put in to e.g. finding new products and can then suggest those products to other customers by mining patterns in your sales.
Classification
In the business of reselling, it’s crucial to gather enough structured data on the items being sold, so that buyers can find what they’re looking for. This can mean manually entering extremely detailed data, a pain point for sellers. Enter image classification: It can help suggest e.g. a car’s make and can automatically pull all available associated data, e.g. engine capacity. The seller only has to review this data instead of entering everything by hand. [1]
Similarly, you might want to utilize communication data (email, social media post) to derive metrics you care about - e.g. percentage of negative interactions, recurring topics, etc.
Forecasting
Forecasting is supposed to improve decision-taking. Maybe you want to manage your supply chain to reduce storage cost - it makes sense to forecast shop sales. The same goes for energy producers forecasting energy consumption to better match production at any given moment. One more example: mining your past project data to get a better estimate of resources to allocate to future projects can be a good idea.
Regression
An example of regression is price optimization. Based on input data you want to predict a number - e.g. house price based on location, number of rooms and area. Or you want to increase your airplane ticket sales based on demand.
Conclusion
Improving business process - either solving pain points or making use of a growth possibility - is a constant challenge and defining projects achieving such improvements is hard. One resource at your disposal is the data available from business processes. I hope this short overview of different types of such data, including examples of how they can be leveraged, will prove helpful.